MicroLearn (2016 - 2019)
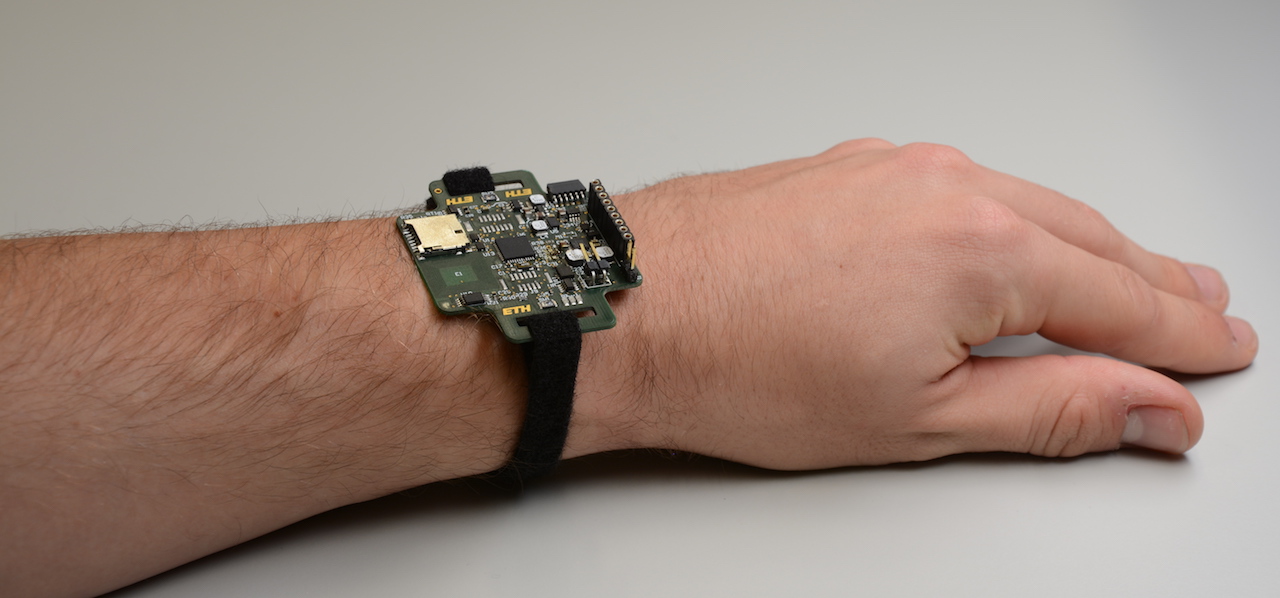
An early prototype of a smart device that can run perpetually with energy harvested from the environment and capture audio and images to detect user activity.
The Micropower deep learning project (MicroLearn) aims to push beyond the current power walls for deep learning and move toward micro-power deep learning. This requires working on algorithms, architecture, circuits as well as design methods for deep learning under extreme constraints: tiny energy buffers (batteries), miniature energy harvesters, low-power and low-cost logic and memory devices. Specifically, the project aims to study and develop the basis for a new 's generation of deep learning engines operating within a power envelope of a few mW. The project’s technical objectives are:
- Reducing the digital computational load, by curtailing the raw digital bandwidth produced by sensors through mixed-signal techniques which mix feature extraction with traditional analog-to-digital conversion.
- Increasing the energy efficiency of digital computational cores with massively parallel near-threshold accelerators, which retain flexibility and high performance on the application domain, while at the same time significantly reducing power with respect to general-purpose parallel instruction-set processors.
- Optimizing deep-learning algorithms to target ultra-low power hardware with limited computational and storage capabilities. Focus will be on robust training of classifiers with reduced numerical precision and weight compression techniques. Semi-supervised and unsupervised techniques for ultra-low power devices will also be explored in this context.
- Demonstrating algorithmic, circuit, and architecture innovations by developing real-life demonstrators of full-system prototypes based on advanced fabrication technologies.